Open access publishing and data sharing, which allows immediate, free, permanent and unrestricted access to scientific results, is increasingly supported by both scientific institutions and funders worldwide. Making data available according to FAIR principles does not mean making data available without any restrictions. The aim is to follow the principle, i.e. to make data accessible:
„…as open as possible, as closed as necessary“
According to this principle, research data should be made publicly available to the maximum extent possible to allow reuse. On the other hand, however, disclosure may be limited, precisely to the extent necessary to protect the rights and interests of the beneficiary, the provider and third parties. These protected rights and interests typically include the right to the protection of personal data, the protection of national security or, for example, the interest in monetising and capitalising on the research carried out by the institution that produced it.
Research data
According to Zákon 130/2002 o podpoře výzkumu, experimentálního vývoje a inovací (Section 2 Definition of Terms, Paragraph 2 letter o), research data means…
“information in electronic form that is collected or generated in the course of research, is used as evidence, or is generally accepted by the research community as necessary to validate findings and results.”
Directive of the European Parliament and Council on open data defines data as:
Research data includes statistics, results of experiments, measurements, observations resulting from fieldwork, survey results, interview recordings and images. It also includes meta-data, specifications and other digital objects. Research data is different from scientific articles reporting and commenting on findings resulting from their scientific research.
FAIR principles:
Research data should fulfill the FAIR principles. These principles describe how data should be processed to make it Findable, Accessible, Interoperable and Reusable. Data should have standard formats, should be described according to disciplinary metadata standards and assigned with persistent identifiers (DOI, handle).
It is not in contradiction with the FAIR principles if access to data is limited with some kind of restrictions (e.g. signing a contract and complying with contractual restrictions, etc. – as long as the restrictions are transparently explained in the DMP).
As an introduction to data management according to FAIR principles, you can use the PARTHENOS Guidelines to FAIRify data management and make data reusable, which summarizes and explains the basic principles in 20 points. The guide contains recommendations for both researchers and archives.
1. To be Findable
The first step in (re)using data is to find them. Metadata and data should be easy to find for both humans and computers. Machine-readable metadata are essential for automatic discovery of datasets and services, so this is an essential component of the FAIRification process.
2. To be Accessible
Once the user finds the required data, she/he/they need to know how they can be accessed, possibly including authentication and authorisation. The best way is to use trustworthy repository (domain specific, institutional or general).
3. To be Interoperable
The data usually need to be integrated with other data. In addition, the data need to interoperate with applications or workflows for analysis, storage, and processing.
4. To be Reusable
The ultimate goal of FAIR is to optimise the reuse of data. To achieve this, metadata and data should be well-described so that they can be replicated and/or combined in different settings. Public licenses are the way how to achieve transparency and predictability for reusers.
FAIR principles in practise
FAIR Principles themselves do not contain any specific technologies or guidelines for implementation in practice, but rather represent a kind of “fairness” in their implementation.
FAIR How-to:
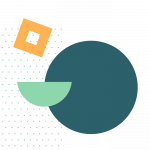
This page will show you clearly how you can make your research data more FAIR through six FAIRification processes (Documentation, File Formats, Metadata, Data Access, Persistent Identifiers, Licensing).
Authored by the Danish National Forum for Research Data Management and supported by the Danish e-Infrastructure Cooperation (DeiC).
Various tools have been developed to serve as guides for data creators to estimate how FAIR their data is. The How FAIR is your research data checklist can be used as a tool to help you evaluate your research data. It is an English translation by Dagmar Hanzlíková of the original English text “How FAIR are your data?” by Sarah Jones & Marjan Grootveld.
If you want to roughly estimate how FAIR your data is, ARDC provides a simple self-assessment tool to find out:
FAIRness of data can also be assessed using tools such as the FAIR Wizard. This is also mainly used to create a Data Management Plan.
The Danish National Forum for Research Data Management has prepared a multidisciplinary How to make your data FAIR page that gives advice on how to FAIRify quantitative and qualitative data.
You can also find this multidisciplinary self-assessment tool on GitHub. It is authored by Kerry Levett, Keith Russell, Martin Schweitzer, Kathryn Unsworth, Andrew White, translated into Czech by Jan Skupa and Petra Dědičová from the Central Library of the BUT, and available online here.
Another self-assessment tool is the 5 ★ DATA RATINGS. The tool implements the FAIR data principles from FORCE 11. Further guides to assess the degree of FAIR data can be found, for example, here.
F-UJI is a web service that evaluates FAIRification of research datasets based on metrics developed by the FAIRsFAIR project.
Life sciences tools
For example, the life sciences ELIXIR Research Data Management Kit (RDMkit) tool is available to provide best practices and guidance on FAIRification of data. This tool is recommended by the Horizon Europe Programme Guide as “a source of guidance and best practice for data management in the life sciences”.
The French Institute for Bioinformatics provides the Life sciences ELIXIR France/IFB FAIR-Checker, a tool to assess your FAIR rate and improve the quality of your digital resources. Data providers can check how FAIR their web resources are. In turn, developers can explore and check the metadata in web resources.
Social science tools
A Data Management Expert Guide is available from CESSDA for social science researchers. This guide can also be downloaded.
Based on Guides for Researchers – How to make your data FAIR – OpenAIRE support page and materials GO FAIR Initiative.
Last updated on June 30, 2025